The COVID-19 pandemic will prove to be a decisive inflection point in healthcare. Not just for the impact it had on patients and providers, but as the moment that broke the status quo economics of the US healthcare industry.
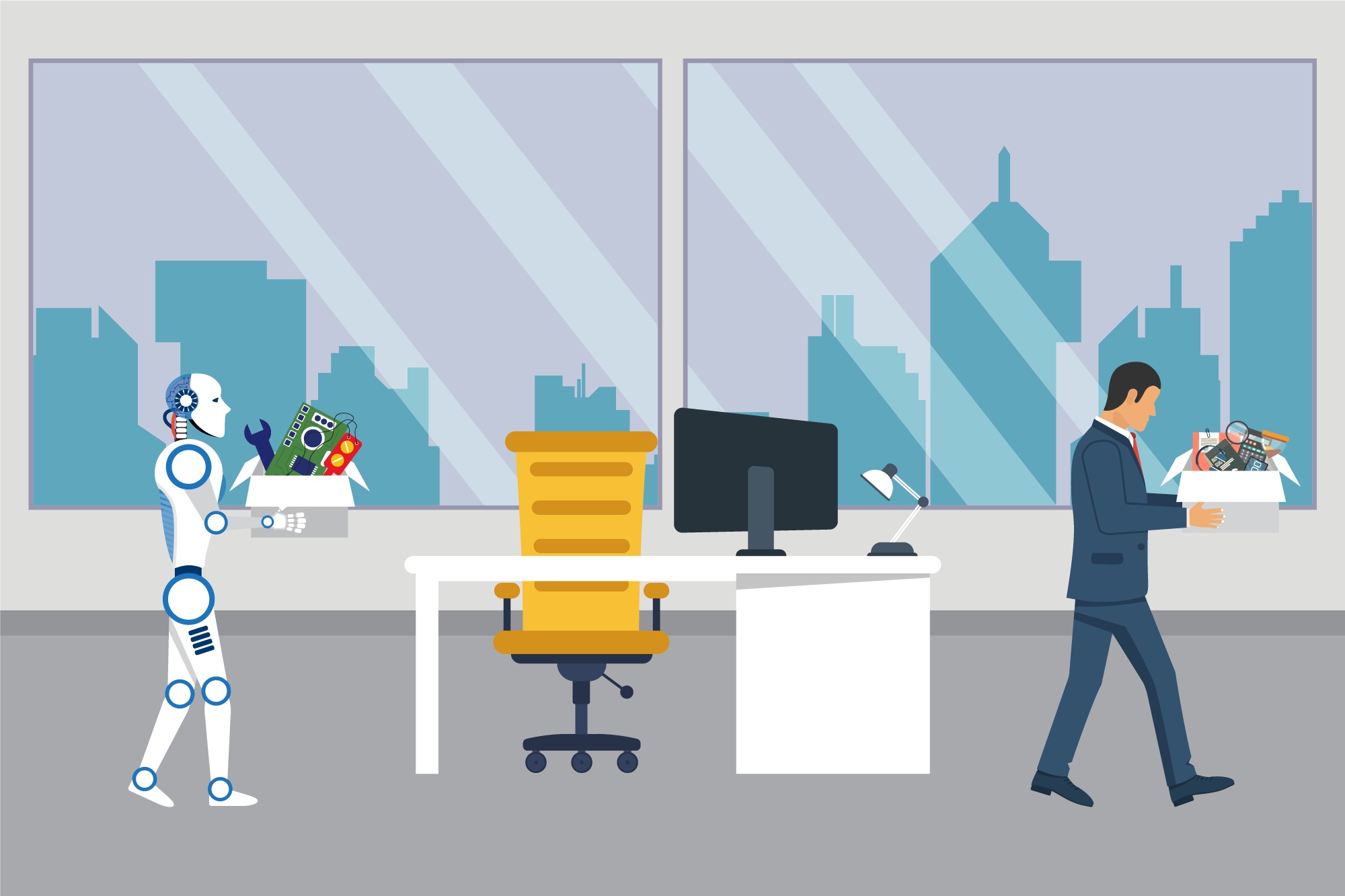
During the pandemic, the digitization revolution that has swept over other industries has finally shown its ability to reshape healthcare. The shift of care from in-person to virtual was previously commonly viewed as technically feasible but culturally impossible. That “impossible” has now happened, and quickly. While trying to serve the community and maintain volumes, health systems just inadvertently proved to disruptors that its “Maginot Line” of high-cost, local monopolies can be beaten.
Payors and employers won’t ignore the potential savings from healthcare digitization. This is particularly true of the largest payor, the federal government, who will emerge from the COVID crisis with debt levels exceeding the period after WWII. Then, faced with increasing healthcare costs and aging on the one hand, and slowing population growth and tax revenue on the other, it will finally have to act.
This will be a big change because for decades, everyone won in healthcare. Some industries and stakeholders did much better than others, but everyone got a share of an increasing pie. There will finally be stakeholders who will lose in healthcare. The question becomes who and why, and the best answer to who and why begins with some key points of market failure within healthcare – starting with the composition of the healthcare workforce.
The healthcare workforce, particularly medium-skilled administrative jobs, has exploded over the last five decades. There has been far more growth of administrative helpers than clinical doers.
Source: Borders, Max, “The Chart that Could Undo the US Healthcare System,” Foundation for Economic Education, April 2015.
Meanwhile, the tools of digital transformation—elastic cloud computing, sensors and other IoT connected devices, big “clean” data and AI/ML tools such as “chat boxes” — are immature in healthcare in part because health systems are busy installing and upgrading last-generation technologies and have been oversold IT “transformation” projects in the past.
The rise of digital health solutions gives competitors a chance to offer consumers and payors a lower priced product through less people and more value – just as Amazon and many others have done. As Aaron Martin of Providence said, “nowhere has the physical pricing point held up once the digital path was introduced.”
Resilient health systems will finally tackle the middle manager “bubble,” making the next logical question: who creates the technology and services to replace them?
Technology companies like Google have invested heavily in digitizing healthcare and should only double their efforts with the provider community under new pressure. However, if healthcare providers effectively outsource their administrative roles to Google, they will become dependent on another technology provider (just as they are with Epic and Cerner) to deliver any patient care.
There is another path – one in which health systems are their own disruptors, where they collectively bring together the intellectual horsepower and financial capacity to invest in essential technologies from a position of strength (ask the last generation of Google, Apple and Microsoft health leaders how strong they felt building into this industry) and before they are undercut by lower cost online players. That is the concept, but practically, what would this new virtual role look like inside a health system day to day?
What would the digital middle manager do?
Observing the utilities and manufacturing industries as models, how might we embrace a digital middle manager for the healthcare space? The new model could:
- Use AI models learning from sentiment analysis, virtual interaction points (e.g. call centers) and in-person volumes across the physical footprint of the system to predict and shape demand across the continuum of care.
- Move from a manual, static demand template to a dynamic, personalized, data-driven model to match each person to the right provider in the right place at the right time. This can be achieved by using existing demand data, as well as historical clinical performance and patient data, to determine who gets seen by which provider and how long that appointment should take. This would evolve past the template view of demand types and the morning review of schedules, where it is often too late to manage demand. This customized model is possible through the scalability of AI and better leverages queuing theory (pooling of demand as much as possible to manage variation) into healthcare settings. A critical part of this approach is to not just better match demand to supply but also divert some demand to chatboxes and other functions which were well demonstrated during the initial COVID-19 surge (e.g., self-diagnosis tools and routing of patients). No other industry tries to put a person on every customer engagement.
- The same data can then also be used to manage beds and support staff levels, from patient transfers to imaging hours. For example, when a hospital is faced with an emergency, instead of the dramatic either-or choice of calling a code and keeping hundreds of staff at the hospital or underservicing patients in-house and delaying discharges, the system could offer bonuses to targeted workers to work specific additional hours. An analogy would be congestion pricing that incentivizes additional Uber or Lyft drivers to enter service. The concept that key pieces of capital and highly trained staff critical to patient throughput are scheduled the same hours every week is repeated in no other service industry. CFOs and CNOs can still exert control, but no longer need multiple chains of people to get them to a decision point.
- That same modeling would apply to clinical areas, not just to predict daily/hourly bed staffing or which units to flex, but also to forecast the right balance of clinical resources from a quality and patient satisfaction perspective. This system would take clinical and enterprise business data (both HR and financial) to understand historical relationships by unit and offer data-driven tradeoffs to guide critical decision-making by nursing and department managers in real time. This will bring actionable answers to often hard and emotional conversations on the floor around hospitalist ratios or nursing staffing/education levels. Also, by having a consistent decision architecture, units will be opened and closed faster to manage demand and costs, without needing to go through rounds of conversations and gatekeepers inside the organization to make that decision. The same logic applies to staff and patient transfers inside the system- creating detailed data-driven admissions criteria.
- Replace coders and quality data abstractors through NLP (Natural Language Processing). Today, hospitals have numerous staff extracting coding for billing, reviewing the process, chasing providers for additional documentation and separate teams. These revenue cycle teams have been internally and externally staffed but still consume millions in overhead at almost every hospital and health system. While using AI is a difficult implementation in a mission-critical function, and getting this right takes significant human and financial capital, the long-term savings is enormous, with synergistic impacts to operational visibility and ability for real-time performance improvement.
- Finally, AI can bring critical logic to one of the industry’s most chaotic processes: patient progression and discharge management. Today, most health systems have case managers shepherding individual patients through the process, often chasing many different clinical staff along the way before securing transportation and post-acute placement for many. Through worklist management for providers and chatbot interactions with patients, we can redirect care managers to focus critical time and attention on both clinical and social determinants of discharge fulfillment.
Through pooling of resources and collaboration, resilient health systems can solve their own costs problems before Google and Amazon simply outcompete them. A few very progressive health systems are beginning to bite off big chunks of this opportunity. For example, Providence just launched Dexcare, and there are promising but narrowly focused AI start-ups like Qventus, and Olive. However, those solutions are designed to eliminate low end work, not the more costly and revenue impactful middle management. This is important work, but incomplete for the nature of the problem, and like EMR vendors, those companies will be largely successful through intercepting – rather than building – health system margin.